
Over the past two centuries, economists, policy makers, and researchers have aspired to “harden” social science. A lot of progress has been made: using randomized, controlled experiments; making “evidence-based” decisions; and generating rigorous data. At the same time, there are limitations to how far the field can go towards becoming a “true science” which involves generalizability, replicability, and prediction.
This is particularly important in social impact, where we need evidence to make decisions related to policy, funding, and programs, so we can solve intractable problems. Some could argue that we have plenty of evidence, based on the sheer volume of published research. According to Google Scholar, there are 5.7 million published studies on “behavior change.” The Social Science Research Network includes 1.7 million studies. Yet publishing articles doesn’t necessarily translate to accessible knowledge or changes in practice.
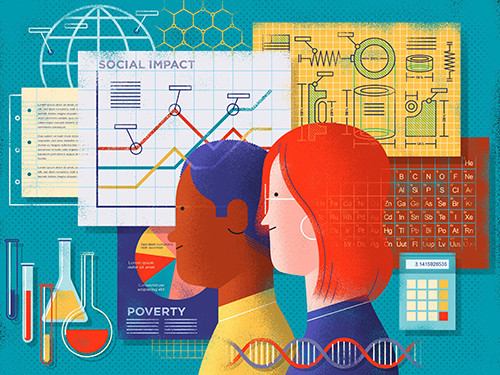
For as much as we know through research, it doesn’t seem to be moving the needle of progress. We looked at spending across the social impact sector; including government, global and domestic philanthropy, and S-themed ESG assets under management; and found that globally we are spending an extraordinary amount of money—roughly $72 trillion annually—making social spending the world’s largest financial market. Yet, according to the United Nations, the world has only made a 3 percent improvement on the Sustainable Development Goals since 2014.
Why do we have so much evidence but can’t seem to deploy it to improve lives? The truth is, it’s very difficult to process and use the information. Duncan Watts, a sociologist at Microsoft Research, critiqued the social sciences’ “incoherency problem” (his term) in the January 2017 issue of Nature Human Behavior:
“You read one paper and then another paper, and it’s got the same words in the title but different units of analysis, different theoretical constructs, entirely different notions of causality. By the time you’ve done a literature review, you’re completely confused about what on Earth you even think. This is not about whether any particular claim can be replicated, right? It’s that collectively the claims don’t make sense.”
Others have made similar points in the context of evidence-based practices, noting how difficult it is to use evidence to predict “what works.” William Riley, the Director of the Office of Behavioral and Social Science Research at NIH, and Daniel Rivera, a professor at Arizona State University, wrote in 2014, “An extensive literature has established the effectiveness of various behavioral interventions for a range of conditions but this literature often fails to isolate the intervention components that are more or less effective. Therefore intervention development largely remains a black box...a process of educated guesses.”
Mark Lipsey, a prominent meta-analyst in social impact, puts it another way: “The true challenge is not, therefore, a lack of knowledge of what works, but rather is in translating the robust body of knowledge into practice.” This illustrates some of the major barriers in this space—not only is it difficult to make generalizable claims about “what works,” it’s also difficult to know why something works, and how to put it to use.
How can we start using all of the information we have to improve lives? This is the goal of a new field we call impact science.
What’s Holding Us Back?
With support from some forward-thinking funders like Google.org, Splunk for Good, and MasterCard Center for Inclusive Growth, we at Impact Genome Project, a public-private initiative focused on creating objective metrics for social impact, mapped the emerging field of impact science, focusing on innovative social impact researchers, evaluators, data scientists, policy makers, and philanthropists. We set out to answer the following questions:
- What are the foundational principles of Impact Science?
- What are the most critical conceptual and practical challenges in the field?
- Who are the key players?
We identified over 130 individuals pushing the boundaries of social impact, including program evaluators, econometricians, academic researchers, machine learning/AI specialists, statisticians, implementation science experts, meta-analysts, and more. Through this work, we identified four barriers that are holding us back:
- The lack of universal, standardized terminology, leading to fragmented and inconsistent evidence;
- The lack of centralized repositories containing structured, “refined” data;
- The lack of market incentives rewarding the use of data to inform decisions that can be linked to capacity limitations in the field to put such data to use; and,
- An academic affinity for prioritizing highly-individualized studies, using methods developed for clinical research, as opposed to more practical—but potentially “lower quality”—evaluations that may better capture real-world conditions.
These barriers limit our field’s ability to be more “scientific” about our knowledge. And this, in turn, limits the ability of social science to solve for external validity and ultimately predict outcomes. As a result, the best that policy makers can do is to rely on lists of previously evaluated model programs that are considered “evidence-based.” This approach constrains the universe of choice for policy makers and doesn’t advance our understanding of why some programs work and others do not. The consequences are real—some experts estimate that less than 1 percent of US government spending on human services programs is based on “evidence of actual impact.”
Solving these challenges is the ambition of impact science.
What Is Impact Science?
The field of impact science is interdisciplinary and builds on what we already know, by organizing that information with greater precision and structure. Impact science uses structured data and probabilistic models to predict the effectiveness of social interventions, and eventually design more effective ones. This is similar to how econometrics uses statistics and modeling to forecast, which in turn informs policies intended to produce better economic outcomes. Using data in this way, social impact practitioners can radically improve program design, make better-informed resource allocation decisions, and ultimately improve the lives of a lot more people for the same or less money.
We identified three conceptual building blocks for impact science: Data standardization; aggregation and synthesis methodologies; and prediction, matching, and benchmarking.
1. Data Standardization
The lack of standardized data is foundationally what’s holding our field back. There is no periodic table of elements for social change, no common genes or chromosomes to sequence the DNA of social interventions, and no universal terminology for elements of social programs. Without these, social impact research remains little more than a compilation of well-documented PDFs. “In emerging fields, you begin to see the development of standards as a good signal that something’s happening there,” Adam Russell, an anthropologist working for the Department of Defense, told Wired in a 2017 article. “We certainly don’t have those standards in social sciences.”
Because of this, researchers and evaluators create their own terminology or frameworks, leading to inconsistency across studies. Defining the data elements of the social sector in a standardized way is an essential, required step in impact science because it allows for the creation of large datasets containing evidence related to program components, implementation, context, beneficiaries, and outcomes. Though large datasets certainly exist in this space already, the difference here is the ability to bring together evidence from across and within multiple sources, including research, policy, and program evaluations.
There are some efforts afoot to standardize key elements of social impact, such as logic models, behavior change mechanisms, and other strategies. Over the past 20 years or so, pioneering researchers including Mark Lipsey, Susan Mitchie, and Bruce Chorpita, among others, have created core component frameworks for fields such as juvenile justice, public health, and education. Others, including Mary Ellen Wiggins at Forum for Youth Investment, and policy makers in The Office of the Assistant Secretary of Planning and Evaluation within Health and Human Services have advocated for the use of core components in government decision-making. These are critical steps forward, but core components are just one piece of the puzzle.
To truly leverage what we know, we must also standardize other data: social impact outcomes, beneficiary characteristics, and program context. Mitchie’s work with the Human Behaviour Change Project is a good example. Mitchie and colleagues developed the Behaviour Change Intervention Ontology—a set of definitions for entities and relationships used to describe behavior change interventions, their contexts, effects, and evaluations.
Our research initiative, the Impact Genome Project, is another example of data standardization. Over the past seven years, we have created standardized taxonomies for 132 common social outcomes, the strategies used to achieve them, and characteristics of beneficiaries and program context. Our taxonomies cover a wide range of areas such as public health, education, workforce development, financial health, social capital, housing, and food security. This standardization allows for apples-to-apples comparisons across programs and studies. This enables aggregation, synthesis, prediction, matching, and benchmarking of data across tens of thousands of research studies, evaluations, and grant reports.
One of the key challenges of standardization is to identify the right conceptual language that is informed by, meaningful to, and usable by all stakeholders. There are also risks if standards are not built to evolve as society and science progress. However, we can take inspiration from other fields that have successfully created and maintained standards (see the NIH MeSH taxonomy of biomedical terms, the NIST standards for physical sciences concepts, and internet protocol standards, to name a few).
2. Aggregation and Synthesis Methodologies
Once standards exist, they can be consistently applied to evidence and other unstructured data. In the social sciences, this is called coding—essentially, applying standards to a (typically) qualitative source and extracting the meaning it holds in a structured way. In impact science, this process can turn any unstructured data—such as program evaluations, PDFs of randomized control trial studies, grant reports—into coded, structured datasets that can be used to analyze outcomes, core components, beneficiary characteristics, and contextual factors. Other sectors have developed data refineries to do just that: make data more valuable and useful. To borrow an analogy from Scientific American, it’s similar to the idea of oil refinement, in that crude oil is not very usable, but refined oil is highly usable for multiple applications. (See also “Does the Social Sector Need An Impact Registry” published in SSIR.)
3. Prediction, Matching, and Benchmarking
The final frontier of impact science is not just structured data, it’s how you use it. Structured data supercharges analytics such as meta-analysis, predictive analytics, benchmarking, and matching. Imagine the possibilities of harnessing the predictive power of data for good, using methods successfully deployed in other sectors like marketing, economics, and even meteorology.
There are a number of organizations—we identified nearly 30 of them—advancing these methodologies. GovLab is looking at innovative ways of encouraging and using open government data for decision-making in developing economies and criminal justice, among other areas. Meta-analysts such as Mark Lipsey and Larry Hedges are pushing forward new methodologies to understand linkages between program strategies and outcomes. Predictive analytics and recommender engines are becoming more widely used to improve social supports and outcomes, for example in child welfare, college graduation, and health care. We’ve also been working on this at Impact Genome Project. In 2020, we published the first taxonomic meta-analysis of childhood obesity prevention interventions in collaboration with researchers from NIH, CDC, and several leading universities, which identified specific intervention components—not full interventions—correlated with positive outcomes.
Impact Science in Practice
When you apply common standards to large amounts of unstructured data, synthesize it, and analyze it, extraordinary breakthroughs become possible. Just imagine being able to…
- Use probabilistic models to estimate the likely impact of a social program or policy before you fund it;
- Use core components to quickly determine how well a program is supported by evidence;
- Tailor programs and policies to specific beneficiaries and contexts;
- Benchmark or compare the cost-per-outcome of one intervention versus another;
- “Gene edit” in-field social programs by adding or removing core components to optimize impact;
- Identify gaps in the evidence base so you can invest in high-yielding research;
- Curate a universal “impact registry” where nonprofits, researchers, and government programs can report their results using a common standard; and,
- Create “outcome markets,” like carbon markets, where governments and philanthropists could “purchase” outcomes directly from nonprofits.
Here’s an example of how impact science works in practice: Imagine you are a funder focused on increasing health equity in your community. Instead of reading through published studies of “gold standard” model programs, you turn to an impact registry, a universal evidence base of studies and funded programs. You can search the registry to precisely identify nonprofit programs by outcomes, beneficiaries served, program strategies, and geography. You can find generic or un-evaluated programs that are deemed “evidence-based” because their program design is based on a meta-analysis of many studies. You can determine how much to invest in a particular program using financial benchmarks that compare similar programs based on their cost per impact. And you can track your investments over time, comparing their impact and costs to industry benchmarks.
Next Steps for Impact Science
Impact science has the power to totally transform philanthropy, government funding, academic research, public policy analysis, program evaluation, management consulting, ESG investing, nonprofit fundraising, and many more adjacent fields. So how do we make it happen?
As with any pioneering effort, it starts with creating a “coalition of the willing” among key stakeholders, including early adopter policy makers, researchers, evaluators, funders, and nonprofit practitioners. The good news is that various workstreams of impact science are already underway with various academic institutions and practitioners. Coordinating the work and centralizing it at this early stage will pay dividends.
Here are three ways we can move the field of impact science forward right now:
1. Create more positive incentives for using Impact Science.
Right now, using evidence and data is largely discretionary and up to individual choice. Programs that use data to improve their effectiveness or impact do not get rewarded financially. Putting the right financial incentives in place—in terms of rewards, not just accountabilities—can help accelerate change.
There has been some legislative progress, like the Digital Accountability and Transparency Act of 2014, the Foundations for Evidence-Based Policymaking Act of 2018, and the Grant Reporting Efficiency and Agreements Transparency Act of 2019. These efforts have elevated the importance of creating open data, using evidence, and standardizing grant reporting. But most of the open data and data standardization legislation focuses on financial data, not impact data. We need legislation that institutionalizes impact data standards, requires the use of evidence and data to maximize grant impacts and mandates impact verification and reporting.
Similar financial incentives can be put in place in state and local government, as well as in the fields of ESG, philanthropy, impact investing, and municipal finance. Other innovative financing mechanisms can be put in place to incentivize the use of data for maximizing returns, such as outcome trading markets, outcomes-based budgeting, reverse auctions, and impact indexes.
2. Build more innovative tools so stakeholders can use predictive data.
Another key field driver is expanding access to impact science. Currently, interacting directly with complex impact data is challenging for anyone outside of academia given the expertise and resources needed to access, extract, synthesize, analyze, and use it. There are plenty of incredible resources for non-academics that summarize what we know, but in most cases, they are not easily customizable and precise enough to be actionable.
The solution is to develop better tools, platforms, and technologies that enable users to dynamically interact with data to answer their specific questions, for their specific context. Think about how 23andme makes it easy for anyone to analyze their genetic profile with a simple cheek swab, or how Turbotax makes it easy for anyone to analyze their taxes using wizard-driven software. Or how Lexis-Nexis or Westlaw makes it easy for lawyers to quickly find the closest matching case law. We need simple, user-oriented tools for the social sector that put the power of impact science into the hands of everyday practitioners.
We also have a responsibility as a field to not repeat the mistakes other sectors have made in terms of ethical generation of, use of, and access to data, AI, and other analytic methods. For example, a 2022 survey of global IT senior decision-makers found that 74 percent were not reducing unintended bias in their AI, and 60 percent had not developed ethical AI policies. The work of Ravit Dotan and the organization We All Count are making headway in the ethical use of AI and data, but this should be top of mind for all of us.
3. More academic focus on impact science methods.
For impact science to work, we must have good quality evidence both from academic researchers and from program evaluators and nonprofits operating on the ground. There are several things we can all do to advance the field. First, leading institutions should adopt a common taxonomy of social impact outcomes and core components so that all new knowledge being generated can be easily incorporated into the wider evidence base. This doesn’t mean we have to throw out everything we’ve already done—there can and should be ways of mapping existing frameworks to a set of universal standards. Stephanie Jones at Harvard University has done something similar for concepts like executive functioning and social emotional learning. Journals could facilitate this by requiring standardized coding for published social impact studies.
Additionally, shifting focus from studies and evaluations with strong internal validity (whether a study supports cause and effect ) to those with strong external validity (whether you can generalize results to other situations) can help us make better use of research evidence when applying it to the real world. And further methodological advancements in research synthesis, prediction, and meta-analysis can bring impact science to the same level of sophistication as other sciences.
We encourage anyone interested in learning more to explore our work at the Center for Impact Sciences at the University of Chicago, headed by Jason Saul and John List, and to follow along with the rest of the articles in this series, which will continue to detail ways that impact science can—and is—happening.
Support SSIR’s coverage of cross-sector solutions to global challenges.
Help us further the reach of innovative ideas. Donate today.
Read more stories by Jason Saul, Heather King & Liz Noble.