How Machine Learning can Contribute to Social Good
Now is the right time for businesses, governments, and non-profits to consider ML applications that go beyond automation and improving bottom lines, and figure out how can they use these innovative technologies to contribute to society.
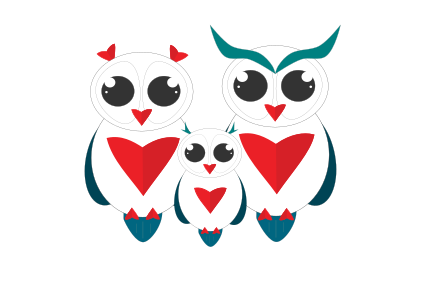
It’s not surprising that machine learning, like any other truly innovative technology, has mostly been viewed in terms of business and economic gains. However, there is a growing recognition that machine learning can be used for social good.
At its core, ML for social good involves using advanced technology to support people in need and strengthen the well-being of our communities. World’s brightest minds have been trying to build ML-based solutions that tackle pressing global issues such as poverty, climate change, education, healthcare, and disaster response. Let’s look at some of the real-life examples of ML for social good.
In this article
Machine Learning Converting Charity Donors
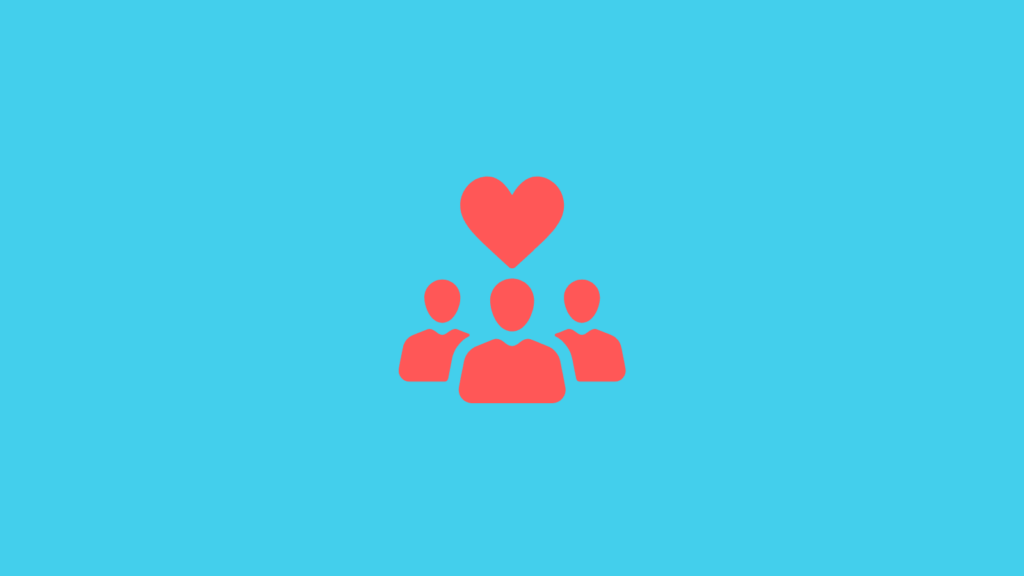
BC Children’s Hospital Foundation, a Canadian non-profit charity that raises money for BC Children’s Hospital, partnered with Deloitte to develop a custom AI model that would help the organization gain deeper insights into donor demographics and economic trends. The model is posed to detect what economic and demographic factors and timing could influence donor behavior. The end goal of the initiative is to turn occasional donors into regular ones, increase donation rates, and reduce donor drop-offs.
Identifying children at risk
Predict Align Prevent is a non-profit organization dedicated to stopping child maltreatment. With the help of advanced machine learning models and geospatial risk analysis, PAP detects areas where child maltreatment is likely to occur in the near future.
It employs ML-based geospatial risk analysis, an advanced modeling technique that detects correlations between human behavior and the surrounding environment. For example, one of the organization’s findings is that 70% of child abuse occurs in just 10% of a city’s area.
Unsurprisingly, child maltreatment is prevalent in areas where substance abuse, domestic violence, family disorganization, and low education levels are prevalent. A victim can file a protective order. But if you’ve been accused, you may need to seek the help of a protective order defense attorney. The model links these factors with geographic data to identify areas where children are more likely to be at risk.
Preventing suicide
A group of researchers from Worcester Polytechnic Institute (WPI) and McLean Hospital in Belmont, which is affiliated with Harvard Medical School, conducted a three-year study utilizing AI to forecast suicide risk in women who suffer from particular trauma-related disorders. The researchers gathered data from 123 women patients at McLean Hospital who had experienced childhood abuse, PTSD, and dissociation disorders and related these factors to previous suicide attempts. Researchers claimed that the developed AI model predicted previous suicide attempts among the examined patients with 83% accuracy.
While detecting suicide risk based on medical history is rather straightforward, advanced natural language processing models can also do that by analyzing one’s writing style. Siddhu Pachipala, a student from The Woodlands College Park High School, developed an ML-based app that can assess suicide risks based on semantics in an individual’s writing with 98% accuracy.
Machine Learning Improving disaster response
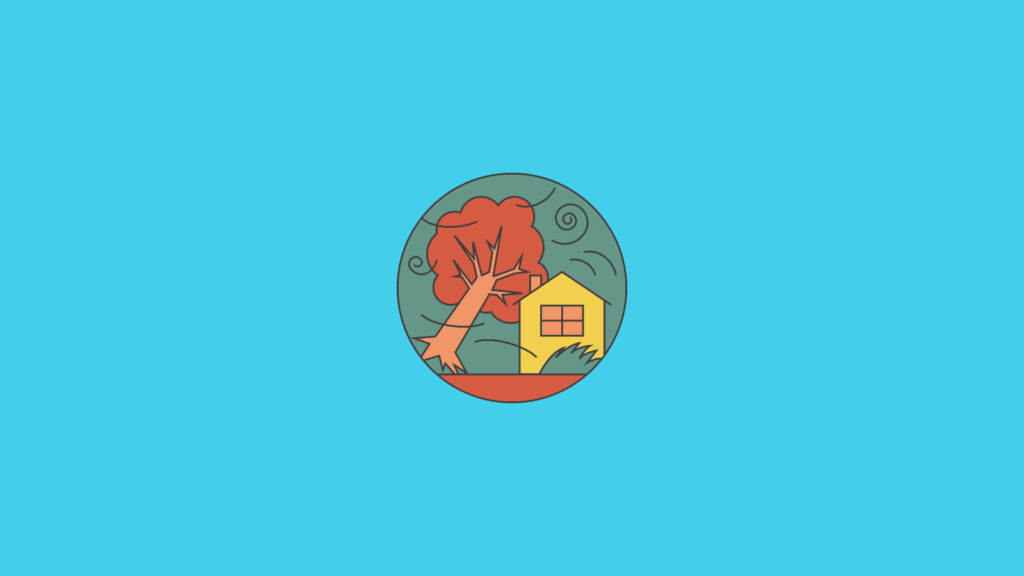
An open-source project sponsored by Pentagon’s Denfense Innovaition Unit called xView uses ML models and satellite imagery to assess infrastructure damage in the disaster location much faster than with traditional methods.
During the recent earthquake in Turkey, xView2 has proven to be an invaluable tool for rescue personnel. Atishay Abbhi, a disaster risk management specialist at the World Bank, claims that xView2 is capable of performing a disaster assessment that would usually take weeks in a matter of hours or minutes. With this AI-powered tool, there is no need for emergency teams to rely on eyewitness reports to detect regions that call for immediate action.
Developers admit that the platform has certain limitations. For example, given that the tool relies on satellite imagery, it is reliable only during the day and in cloudless weather.
Measuring gentrification
Gentrification is a widespread phenomenon plaguing many urban areas around the world, the US in particular. It negatively impacts demographics and local culture and destroys communities. Conventional methods for measuring gentrification are rule-based and have become rather outdated as it’s possible to measure neighborhood change only after it occurs, making all the consequent efforts futile.
IBM has partnered with Urban Institute to develop a new ML-based method to accurately track local neighborhood change based on data from a number of sources, including US Census, Zillow, and the Housing Choice Voucher program. The new approach showcased a significant enhancement over the precision of earlier rule-based methods, raising accuracy from 71% to 74%. While the improvement may seem incremental, further model enhancement will most likely increase its precision.
Helping underserved communities
Wadhwani AI is an independent nonprofit institute that develops AI-based solutions for underserved communities in developing countries. One of its initiatives is CottonAce, a free AI-powered advisory platform for cotton farmers in India. The system assists farmers in safeguarding their crops by identifying the optimal timing and amount of pesticides to apply. Farmers install the CottonAce app, upload a photo of pests, and the embedded AI model determines the level and type of infestation and generates a set of suggestions. Importantly, the app can work offline. In 2022, the app has been used by more than 30000 farmers across 6 Indian states.
Profit is not the end game
As the abovementioned projects demonstrate, ML shows a lot of promise when applied in socially relevant initiatives such as child protection, suicide prevention, disaster response, gentrification monitoring, and many more. However, the concept of using ML to tackle some of the world’s most important challenges rarely yields apparent economic benefits, which remains the biggest barrier to its widespread adoption.
On the other hand, it’s time for business leaders to realize that in our day and age, return on capital is not the only economic goal they should strive for. For example, helping underserved communities to get a better insight into their agricultural processes will inevitably improve water and energy preservation, which, while indirectly, makes a tangible economic impact.
Now is the right time for businesses, governments, and non-profits to consider ML applications that go beyond automation and improving bottom lines, and figure out how can they use these innovative technologies to contribute to society.
FAQ
What is machine learning, and how can it contribute to social good?
Machine learning is a subset of artificial intelligence that enables computers to learn from data and make predictions or decisions without being explicitly programmed. It can contribute to social good by analyzing large datasets to identify patterns and insights that can inform decision-making and address pressing social issues.
In what ways can machine learning be applied to healthcare to benefit society?
Machine learning can be applied to healthcare in various ways, such as predicting disease outbreaks, diagnosing medical conditions, personalizing treatment plans, optimizing hospital operations, and discovering new drugs and treatments, ultimately leading to improved patient outcomes and public health.
How can machine learning help combat climate change and protect the environment?
Machine learning can help combat climate change and protect the environment by analyzing environmental data to predict natural disasters, optimize energy consumption, monitor air and water quality, identify deforestation and wildlife habitats, and develop more sustainable agricultural practices.
What role does machine learning play in improving education and accessibility to learning resources?
Machine learning can improve education and accessibility to learning resources by personalizing learning experiences, automating administrative tasks for educators, detecting learning disabilities early, providing adaptive tutoring systems, and enabling access to educational content for individuals with disabilities.
How can machine learning contribute to public safety and crime prevention?
Machine learning can contribute to public safety and crime prevention by analyzing crime data to predict hotspots and patterns, identifying individuals at risk of criminal behavior, optimizing police patrol routes, detecting fraudulent activities, and enhancing cybersecurity to protect against cyber threats.
What are some examples of machine learning initiatives focused on poverty alleviation and economic development?
Examples of machine learning initiatives focused on poverty alleviation and economic development include microfinance lending platforms that use predictive analytics to assess creditworthiness, job matching algorithms that connect job seekers with employment opportunities, and financial inclusion projects that provide access to banking services for underserved communities.
How can machine learning help improve access to clean water and sanitation in developing countries?
Machine learning can help improve access to clean water and sanitation in developing countries by analyzing water quality data to detect contaminants, optimizing water distribution systems to minimize waste, predicting waterborne disease outbreaks, and developing innovative sanitation solutions for remote areas.
What role does machine learning play in disaster response and humanitarian aid efforts?
Machine learning plays a crucial role in disaster response and humanitarian aid efforts by analyzing satellite imagery to assess damage and prioritize relief efforts, predicting population displacement and resource needs, coordinating logistics and supply chain management, and facilitating communication and coordination among response teams.
How can machine learning support mental health and well-being initiatives?
Machine learning can support mental health and well-being initiatives by analyzing social media data to identify individuals at risk of mental health issues, providing personalized mental health interventions and therapy recommendations, and developing chatbots and virtual assistants for mental health support and counseling.
What ethical considerations should be taken into account when deploying machine learning for social good?
Ethical considerations when deploying machine learning for social good include ensuring transparency and accountability in algorithms, protecting user privacy and data security, addressing bias and fairness in AI models, promoting inclusivity and accessibility, and fostering collaboration and community engagement in decision-making processes.
How can machine learning be leveraged to address food insecurity and hunger?
Machine learning can be leveraged to address food insecurity and hunger by optimizing food distribution networks, predicting crop yields and agricultural productivity, identifying food deserts and areas with limited access to nutritious food, and developing sustainable farming practices to increase food production.
What impact can machine learning have on wildlife conservation and biodiversity preservation?
Machine learning can have a significant impact on wildlife conservation and biodiversity preservation by analyzing wildlife tracking data to monitor animal populations and migration patterns, detecting illegal poaching activities, identifying endangered species and habitats, and developing predictive models to guide conservation efforts.
How can machine learning assist in disaster resilience and infrastructure planning?
Machine learning can assist in disaster resilience and infrastructure planning by analyzing geospatial data to assess vulnerability to natural disasters, predicting infrastructure failures and maintenance needs, optimizing urban planning and land use, and developing early warning systems for natural hazards.
What role does machine learning play in fostering financial inclusion and reducing poverty?
Machine learning plays a crucial role in fostering financial inclusion and reducing poverty by analyzing financial transaction data to assess creditworthiness, providing access to microfinance and peer-to-peer lending platforms, developing mobile banking and payment solutions for underserved populations, and enabling access to financial education and literacy programs.
How can governments, nonprofits, and businesses collaborate to harness the potential of machine learning for social good?
Governments, nonprofits, and businesses can collaborate to harness the potential of machine learning for social good by sharing data and resources, funding research and development initiatives, promoting knowledge sharing and capacity building, and establishing ethical guidelines and standards for the responsible use of AI technologies.
Improve your Marketing with the Power of AI
See how you can start with AI Marketing and reach your goals faster than ever before. Check out the Tips, Strategies, AI Tools, Masterclass, Courses, and Community. Unleash the true potential of your brand with the help of AI.